Status quo in Industrial Quality Control
Companies’ claim to remain competitive with high-quality products could fail due to the degree of automation of their quality controls at the end of the production lines. This is where most work is still done manually. Although automation solutions such as machine vision do exist, the high programming costs significantly reduce the cost-effectiveness of such systems. Moreover, the approach is not suitable for every scenario.
While a shortage of skilled workers, increasing demand for quality and precision with a growing number of component variants, and cost pressure determine the entire company, manual quality controls appear to be the worst possible method. They are not only time-consuming and cost-intensive but also prone to errors. This is simply because inspection accuracy depends on factors such as the employee’s qualifications, daily form, and motivation. External conditions such as the correct lighting also influence the results. For all these reasons, it makes sense to automate quality control processes as completely as possible. Here, artificial intelligence in the form of machine learning can be worthwhile. In combination with the appropriate hardware, corresponding models are able to identify defective products quickly, efficiently, and extremely precisely.
Limits of classical machine vision
Machine vision systems have been used for some time to automate inspections. These systems essentially consist of cameras and corresponding machine vision software. As good as this approach is for measuring exact distances or checking for completeness, it also has weaknesses. First, it causes a high programming effort because an exact rule must be defined for every possible defect on a component. Especially in the case of variable defects on the surface of components, such as scratches or cracks, classical machine vision systems reach their limits because it is difficult to define a rule for every manifestation and position of a defect. This is also the reason why machine vision systems have a high pseudo error rate for surface defects. This results in too high reject rates, which calls into question the overall cost-effectiveness of the systems.
How deevio solves this problem
deevio automates visual inspections in production. The core product is an artificial intelligence-based machine vision software that helps manufacturing companies automate their still manually performed quality inspections or significantly improve their existing machine vision systems. deevio supplies customers with a camera system tailored to the individual application, which can then be integrated into the respective machine environment via a PLC connection.
At this point, it is important to understand how machine learning and artificial intelligence lead the way for new technologies and how deevio applies them to change quality control processes.
Machine learning (ML) is a sub-discipline of artificial intelligence that allows computers to learn from example images. For example, by showing the ML software images of defects on components in various degrees and positions, it can generalize the defects and recognize them on components not yet seen. This makes it possible to map a high variability of defects and standardize the evaluation of defects. These features make machine learning an ideal technique for industrial quality control.
Deevio applies ML algorithms to industrial quality control. The goal is to automate visual inspection at the end of the production line. The system is essentially composed of two components: hardware and software. These include industrial special cameras and a mini-computer, as well as the machine learning model for defect detection.

Case study
To get a better understanding of how the technology works, it is necessary to take a look at one of the projects deevio has completed. Deevio has worked together with a manufacturer of die-casting aluminum parts for the automotive industry. Among other things, the company manufactures throttle bodies, which were manually inspected after the casting process for defects such as flaking, pores, or pressure marks. These surface defects, which are typical for die-casting products are difficult to recognize for traditional machine vision systems. As the defects have a big variety of locations or sizes, it is difficult to establish a rule.
Considering the variety of defects and the challenging casting surface, deevio designed a camera setup, resulting in all defects becoming visible on the images. The handling of the butterfly valves is then done by a robot arm, which guides the components in three different positions under the camera and thus can check all sealing surfaces to be inspected. After the proper setup of the hardware, the images could be taken and the AI model created to detect the defects. No complex test code is required for the creation of the AI model; sample parts with the defects to be tested are sufficient here. deevio takes pictures of these, marks the defect on the picture together with the automotive supplier, and can then carry out the first tests after just a few hours.

Providing and evaluating sample parts is the only thing deevio’s customer had to do here.
In doing so, deevio not only shows that a throttle valve is defective but also where in the image the defect is. This allows the customer to keep statistics on the defects that occur and improve the production process.
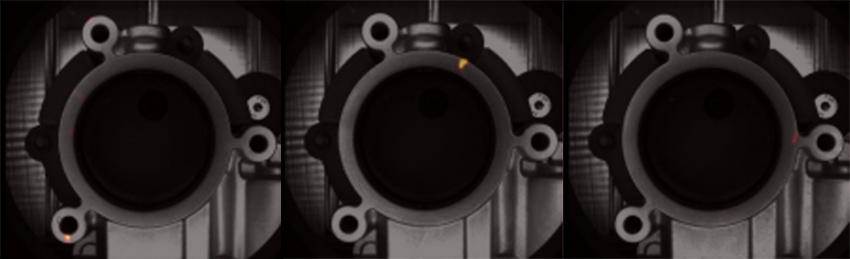
Challenges we encounter and future outlook
If we set up a machine vision system, the goal is to get the pseudo error rate under 1%. To be able to reach this goal, deevio has to create an accurate AI model which makes the correct predictions. For this to happen the most important component is the data. Getting the right amount and especially correctly labeled data is one of deevio’s biggest challenges. Especially, in the case of industrial automation, the data set sizes are much smaller, as it is not possible to collect huge amounts of data. Therefore, what’s important is not the quantity of data, but the quality. If the quality is not right, the project will not be successful. In order to achieve a widespread AI deployment in manufacturing, our focus must shift from high quantities of data to high-quality data.
So how can we ensure that we have high-quality data sets? deevio’s solution was to develop a labeling tool, where non-programmers can easily label images. Manufacturing experts are the ones who have knowledge of their products and the factory. They are able to determine if the collected data is of high enough quality. It is important to make AI technologies easily accessible and usable in order to maximize the accuracy and quality of the learning data. After the acquisition of sample images, the respective manufacturing experts should label the images, locating and identifying the defects on the parts. Naturally, different experts may have different opinions. Therefore, it is advised to create a defect catalog that specifies various possible defects, so that future quality inspectors have guidelines on how to label data correctly and improve the final system performance.
