SiMa.ai is a machine learning software company with purpose-built hardware, offering a full stack platform for customers to deploy artificial intelligence and computer vision at the edge. The company’s goal is to make artificial intelligence and machine learning more accessible for ML developers and embedded engineers building at the edge. SiMa.ai was founded to address stagnant software and hardware innovation, as the technology to effectively power robotic platforms, security systems, defense applications, automotive devices, and other machines has drastically fallen behind what today’s devices require.
Interview with Alicja Kwaśniewska, Senior Principal ML Architect at SiMa.ai
Easy Engineering: A brief description of the company and its activities.
Alicja Kwaśniewska: Our chip optimizes software and hardware to save energy without sacrificing performance in the low-powered devices that are prevalent across industries being utilized at the edge. We developed our SiMa Machine Learning System-on-Chip (MLSoC) to be compatible with multiple open source and existing legacy applications, so our customers don’t need to write new applications or learn new coding languages to support machine learning.
E.E: What are the main areas of activity of the company?
A.K: SiMa.ai makes it possible for customers to AI-enable their products and services with a purpose built machine learning platform that fuses together hardware and software. This is known as the MLSoC.
SiMa.ai’s Palette software offers a low code, integrated environment for edge ML application development — where customers can effortlessly create, build and profile their solutions. In the past, silicon makers have created one-size-fits-all chips that force innovators to learn how to code their programs to match the chips they are buying. We eliminate this learning curve with Palette, so compiling and evaluating any ML model, from framework to silicon, can be done in minutes. Simply put, we make ML deployment to the embedded edge more accessible.
Customers use our Palette software with our MLSoC silicon and boards, purpose-built for edge ML applications to provide a unique combination of industry standard interfaces, central processing unit (CPU) and specialized processing blocks, such as image, signal, and machine learning accelerators, all in a single SoC.
E.E: What’s the news about new products?
A.K: In June, we entered full production on our SiMa Palette software, MLSoC and boards. Most recently, we announced the launch of Palette Edgematic, a no-code approach to creating, evaluating and fine-tuning ML applications from anywhere in the world. We continue to update and add new features and capabilities to our production Palette software, with our latest release incorporating advanced quantization techniques, python based pipeline creation for quick proof of concepts and multi-camera streaming support of PCIe.
Today, it’s obvious that companies need to embed artificial intelligence into their products and services but developing that is easier said than done. Many companies are not resourced to hire or train a specialist to add AIML to their devices, or they don’t have the time to spend months evaluating and deploying ML applications. With Palette Edgematic, we are giving any individual or organization the power to get started in evaluating ML applications to fuse AI into their products or services within minutes.
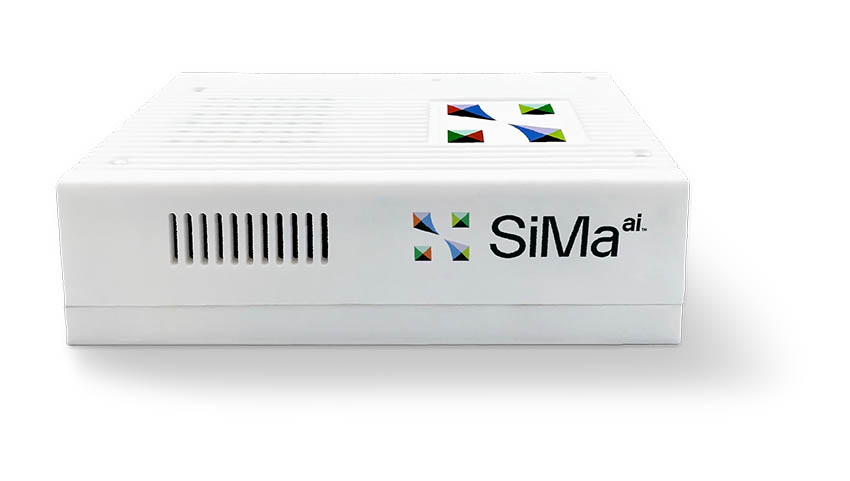
E.E: What are the ranges of products?
A.K: SiMa MLSoC: MLSoC delivers high-performance machine learning for embedded edge applications. Built on 16nm technology, the MLSoC’s processing system consists of DSP for image and signal processing, coupled with dedicated machine learning acceleration and high-performance application processors. Surrounding the real-time intelligent ML processing are memory interfaces, communication interfaces, and system management all connected via a network on chip (NoC). Our innovations in data handling allow us to achieve superior performance at the minimal power consumption, beating rival companies at 8 nm geometry. This means our silicon roadmap can take advantage of Moore’s law and continue leadership as we move to smaller geometries.
Palette Software: Our Palette software addresses ML developers’ steep learning curve by avoiding the arcane practice of embedded programming. Palette is a unified suite of tools, functioning much like an ML developer’s familiar cloud equivalent environments, with push button software commands to create, build and deploy on multiple devices. Palette can manage all dependencies and configuration issues within a container environment while securely communicating to the edge devices. This approach still enables ML programmers with flexibility to create high performance solutions without resorting to low level optimization of the embedded code.
Palette Edgematic: An extension of Palette, Edgematic is a free visual development environment designed for any organization to get started with and accelerate ML at the edge. Palette Edgematic enables a “drag and drop,” code-free approach where users can create, build and deploy their own models and complete computer vision pipelines automatically in minutes versus months, while evaluating the performance and power consumption needs of their edge ML application in real time.
MLSoC™ Boards:
MLSoC Evaluation Board: Standalone MLSoC evaluation board configuration to explore the full capability of our MLSoC bundled with a one year Palette software license.
MLSoC Developer Board: A compact, cost effective standalone developer board that can work on the bench or in a PC, based on our HHHL Production board, bundled with a 6 month Palette software license.
MLSoC Dual M.2 Eval Development Kit: Deeply embedded MLSoC module configuration to explore the performance of our MLSoC bundled with carrier card and our Palette software.
HHHL Production Board: PCIe board for edge ML server application, limited software included.
Dual M.2 Production Board: PCIe module for deeply embedded, space constrained applications, limited software included.
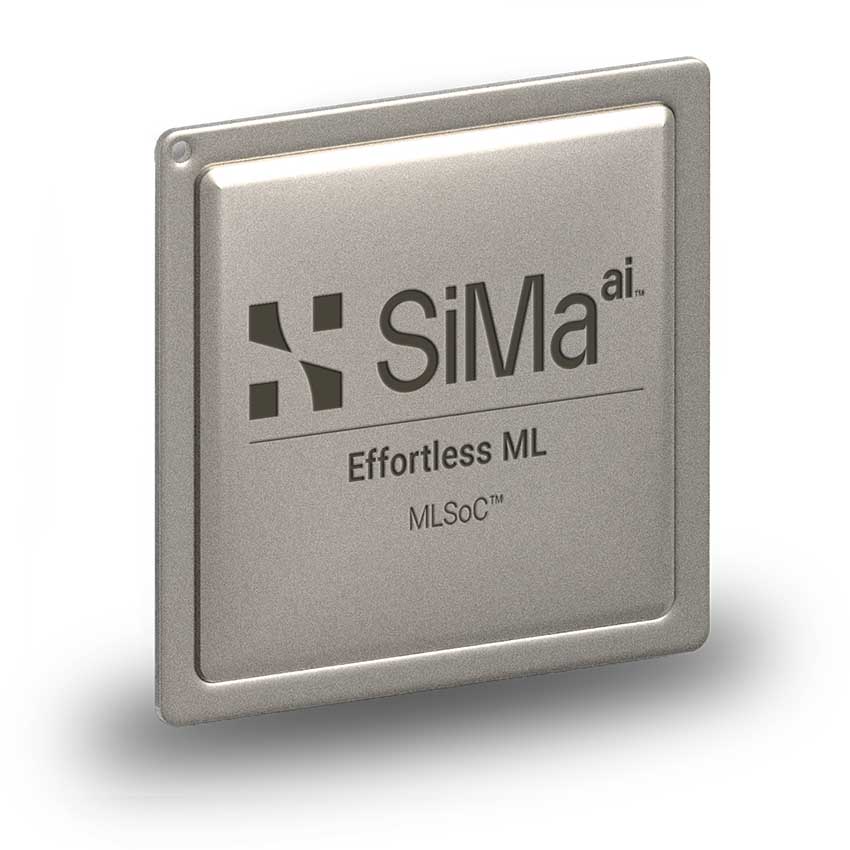
E.E: At what stage is the market where you are currently active?
A.K: In just the past year, the explosion of generative AI capabilities, services and startups has cemented the notion that humans and AI will be forever paired (just as the introduction of the iPhone in 2007 led to humans and machines being forever paired). The launch of innovations like OpenAI’s ChatGPT has made it more and more evident that industries are ripe for AI transformation. In recent years, one of the most underserved markets is computing solutions tailored specifically for edge applications. The fact is, there are countless edge devices in this market – from autonomous vehicles to robotics and drones – that either have yet or haven’t seen a meaningful architecture update in decades. And the race for GPUs for AI workloads is a great illustration of the problem. Those GPUs work, but they aren’t built for the task at hand – that disconnect drives costs higher while hurting both power, efficiency and performance for AIML at the edge.
The embedded edge is a $40B underserved market annually when it comes to computing solutions. That number is set to grow exponentially over the next few years, as AI use cases expand and growing concerns of privacy and security lead companies to keep more data localized on their own devices. That convergence of the growing demand for AI’s application across fields and industries while at the same time needing to address the power and performance restrictions of the edge means the need for purpose-built architectures will only grow. In addition, we believe that software flexibility and a new normal of ease of use for AI deployments will be paramount. That’s exactly what we’re building – the first production generation of our boards was launched earlier this year to help customers easily onboard their AIML to the edge, and are already iterating on what’s coming next.
E.E: What can you tell us about market trends?
A.K: General artificial intelligence is the topic du jour or year rather, and one that has pushed chip developers into the limelight as we demonstrate how we can bring this technology to virtually every application. The demand for low-power, low-latency solutions will only become more integral and prominent as new computing paradigms are required amongst innovators, especially as they work to automate across business-essential tasks. In addition, the need for more sustainable computing will be front and center as a result of sustaining power and energy hungry algorithms. Some estimate that up to 20% of the world’s total power will go to computing by the end of the decade unless we find a different way to power computing.
Our purpose-built machine learning platform optimizes hardware and software to increase performance and save energy. These capabilities will only become more attractive to companies trying to transition from high-powered cloud computing to edge solutions. We’ll start seeing the hype around AI technologies come to light in more concrete and practical ways in 2024, with the technology influencing everything from smarter manufacturing with automated quality inspection to drones that find and transport medical supplies to remote locations.
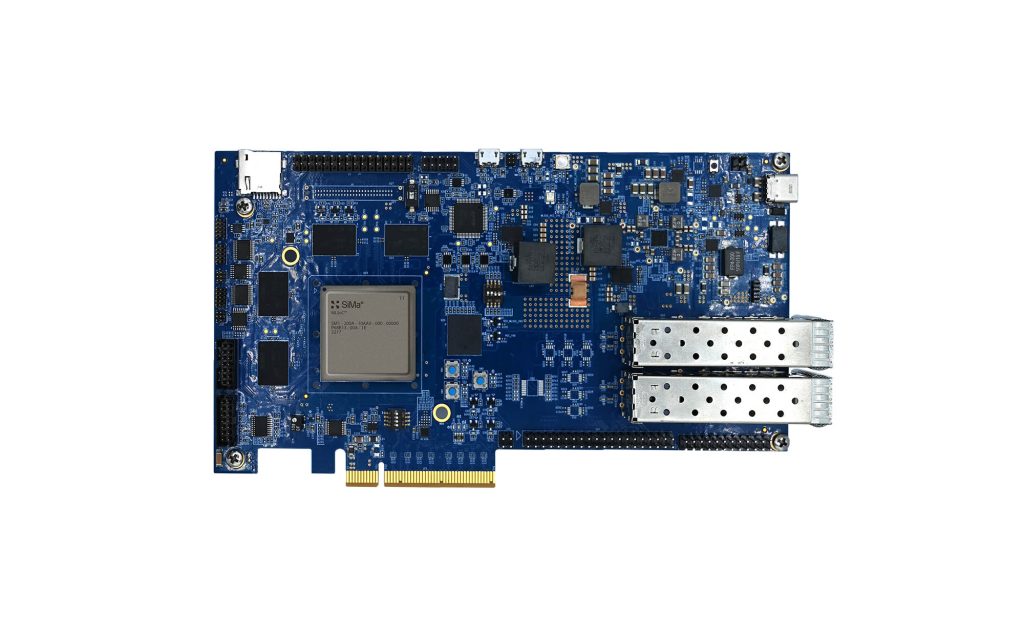
In addition, we will see an increased need for handling multimodal model architectures evolving at a rapid speed. That requires future proof software-hardware co-designed platforms that can handle higher memory demands of such solutions, make sense of data coming from various sensors and allow the flexibility to keep up with the pace of innovation happening among ML researchers. However, this is really needed for solutions to start ‘using all senses’ for understanding the world vs. vision or text only, as we’ve seen before.
Edge is crucial to enable such innovations while mitigating some concerns appearing along with GenAI, LLM, LMM solutions. Data privacy is a big issue of using such solutions. More and more companies are concerned about how to ensure that foundation models do not expose their private data. Deploying such systems locally will naturally solve such problems, allowing for a full control of your information.
E.E: What are the most innovative products marketed?
A.K: We have demonstrated twice this year through MLCommons’ MLPerf suite of benchmarks that the SiMa.ai MLSoC leads in embedded edge performance and power efficiency based on frames/second/watt, outperforming Nvidia in particular. MLCommons’ MLPerf benchmarks are largely regarded as the Olympics of machine learning or the gold standard in comparing like for like system performance of AI capabilities.
In April, we outperformed Nvidia in both performance and efficiency in power consumption. In this benchmark, the ResNet-50 computer vision model was used with the SiMa.ai MLSoC to test performance and power. This is the first time a start-up was able to unseat Nvidia in computer vision with higher frames per second performance and higher frames per second per watt efficiency in the edge category.
Again, this September, for a second time we surpassed performance for computer vision while also demonstrating up to 85% greater efficiency compared to Nvidia and outperformed ourselves, showcasing a 20% improvement in the MLPerf Closed Edge Power category.
At the same time, we made exciting progress with customers – a single SiMa.ai MLSoC chip can provide 60 frames-per-second real-time processing of complicated pipelines based on multiple neural networks and computer vision algorithms with less than 15 watts of power consumption, delivering a superior performance for an entire customer application. This deployed in the real world looks like a major aerospace and defense customer improving its detection, decoding, and assistance in their automated takeoff and landing system. Ultimately in the field, this customer saw a nearly 15X improvement over competitors when using our chip with their computer vision technology.
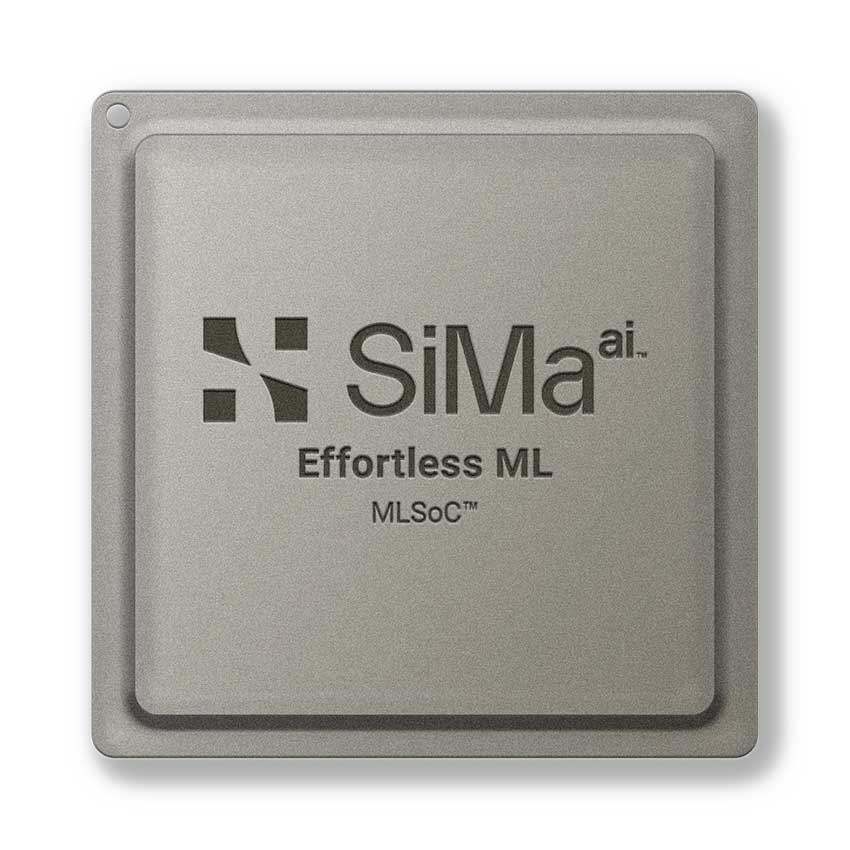
E.E: What estimations do you have for the rest of 2023?
A.K: Many of the technology shifts we’re eyeing in 2024 have already started taking shape. One tailwind in particular is the ongoing movement from legacy hardware buyers to prioritize software flexibility. Today’s innovators realize that the hardware designed for the cloud won’t work for tomorrow’s robots, drones, and factory floors – technology that has to be operated at the edge and cannot afford off-device latency. SiMa.ai fills that gap for companies that want to utilize the full potential of their hardware, regardless of their software stack, and we expect to see more demand from customers and prospects through the end of the year and beyond in 2024 for that reason.
In 2024, we also foresee a proliferation of localized generative AI services powered by smaller, more fine-tuned models. As open source and use case-specific AI models rise, we’ll see companies offer generative AI services focused on specific functions. This will transform technical workflows, to be sure, but will also begin to impact individual cities and municipalities as local governments begin to experiment. Real-time transit information, improved event and restaurant recommendations and reduced traffic will mark just a few of the many positive changes that could come as a result in 2024.
AI systems will also start to become more autonomous. So far, they’ve been using past data with the ability to quickly adapt and respond to changing conditions and new knowledge. We will see more and more systems utilizing a combination of past memories with current observations obtained by interacting with environments to adjust their behavior to specific domains and scale to new tasks. We believe our MLSoC platform and Palette software will drive such innovations at the embedded edge to offer the quickest deployment and real time execution of cutting-edge AI solutions.